CDISC and ADaM: Data Standards Shaping Market Access Success
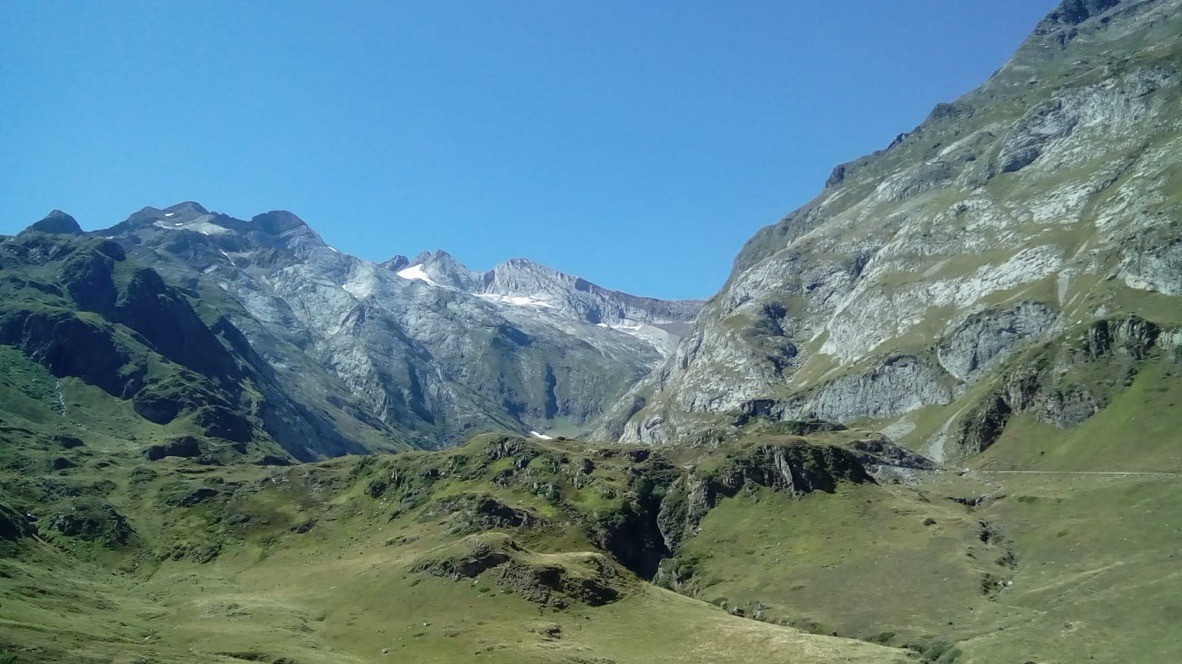
A while ago, a colleague from Market Access asked me: “Why are we investing so much time in ADaM datasets? For HTA submissions, we only need the final results, right?” My answer: Because well-structured ADaM data are the foundation of credible analyses.
As a biostatistician, I’ve seen firsthand how the structure of clinical data directly impacts how effectively we can communicate a therapy’s clinical value and economic value. CDISC standards – particularly ADaM – build the bridge between clinical research and Market Access, supporting everything from HTA submissions to real-world evidence studies and health economic models.
What are CDISC and ADaM?
CDISC (Clinical Data Interchange Standards Consortium) is a global initiative that develops data standards for clinical research. These standards – including CDASH (data capture), SDTM (study datasets), and ADaM (analysis datasets) – ensure that clinical data are consistently structured. Since December 2016, the FDA has required standardized clinical datasets , making it easier for reviewers to understand and verify study results across sponsors .
ADaM (Analysis Data Model) defines how analysis-ready datasets should be structured:
- It provides derived variables needed for statistical analyses.
- It ensures full traceability back to the source SDTM data .
- It simplifies the generation, reproduction, and review of analyses .
Some important types of ADaM datasets include:
- ADSL (Subject-Level Analysis Dataset) – One record per subject, containing demographics, treatment assignment, population flags, and key dates .
- BDS datasets (Basic Data Structure) – Multiple records per subject, one per time point or parameter . Examples: ADTTE (Time-to-Event analyses), ADLBC (Laboratory tests), ADVS (Vital signs), and ADAE (Adverse events).
In short, ADaM is not just about structure – it’s about making clinical analyses transparent, reproducible, and trusted.
Why Standardized Data Matters for Market Access
Once a therapy is approved, the real challenge begins: convincing payers and HTA bodies of its value. Market Access and Health Economics teams heavily rely on clinical study data to demonstrate effectiveness, safety, patient-reported outcomes, and treatment persistence.
Here’s why standardized CDISC/ADaM data matter for Market Access:
- Less rework: With analysis-ready ADaM datasets, analysts can immediately generate new outputs for HTA or payer requests, without extensive data wrangling.
- Cross-functional consistency: Biostatisticians, medical writers, and health economists all work from the same well-defined datasets and derived variables – minimizing inconsistencies across submissions.
- Traceability builds trust: Every endpoint can be fully traced back to the source data , enhancing the credibility of HTA submissions.
- Better data integration: Standardized datasets are easier to combine with real-world data for external comparisons or cost-effectiveness modeling .
In short: Strong ADaM foundations allow teams to quickly answer new questions with robust evidence, without having to reinvent the wheel.
ADaM in Action: Example – Time to Treatment Discontinuation
Let’s walk through a practical example relevant for Market Access: We want to analyze how long patients stay on a new therapy before discontinuation – a key metric for cost models and payer discussions.
In a CDISC-compliant setting, we would create an ADTTE dataset (Time-to-Event analyses) containing one or more records per patient. For example, a record for the endpoint “Time to Treatment Discontinuation” would include:
- PARAM = “Time to Treatment Discontinuation”
- AVAL = 180 (the number of days until discontinuation)
- CNSR = Censoring indicator (1 = censored, 0 = event occurred)
- ADT = Date of event or censoring
With these structured variables, we can easily generate a Kaplan-Meier plot showing therapy persistence, calculate the median time to discontinuation, and derive hazard ratios if needed.
Such analyses provide critical inputs for HTA submissions:
- If patients stay longer on treatment, drug costs increase but so might the clinical benefit.
- Conversely, early discontinuations could imply lower therapy costs but weaker health outcomes.
Similarly, patient-reported outcomes (PROs) like quality-of-life scores can be structured in ADQS (Analysis Dataset for Questionnaires). Analysts can then directly calculate changes from baseline or determine clinically meaningful improvements, which feed into health economic models like QALY (Quality-Adjusted Life Years) calculations.
Thanks to ADaM, these analyses are not only faster but also fully transparent and reproducible – which is crucial when defending a therapy’s value to critical stakeholders.
Thanks to Manuel Pfister Jana Spinner and Laura Jarassier for these insights!